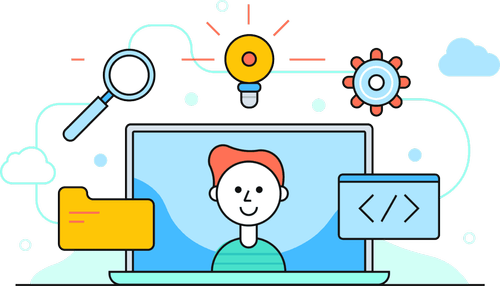
Machine Learning Operations
What is MLOps? If you read it as one word, it kind of sounds like a new alien species: Mlops from Xiban7. But if you stop being silly and read it as M-L-Ops, as in machine learning operations, it’s just as out of this world.
MLOps is all about best practices and continuous improvement in machine learning. As companies continue to implement ML—on our social media feeds, in self-driving cars, and out in the oil fields—there have to be processes in place to monitor and improve ML models.
MLOps is another “ops” in a long line of them:
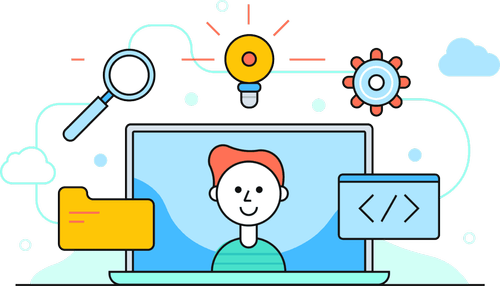
From CNVRG.IO
DevOps (development operations)
AIOps (artificial intelligence operations)
DataOps (this one seems obvious at this point)
And that’s just the tip of the iceberg as combinations like DevSecOps (development, security, and operations) are being coined.
But all of these ops have a purpose: to put a focus on monitoring, managing, and improving each discipline across the lifecycle of use. You can have machine learning with MLOps, but not at scale or with confidence in quality.
As machine learning is implemented in more and more enterprises, development teams will need MLOps in place.
That’s just human learning.